Safe urban futures: exploring the nexus between urban safety and quality of life in South African cities
Researcher: Lerato Tlale, University of the Witwatersrand, Johannesburg
Supervisor: Helen Sarah Robertson, University of the Witwatersrand, Johannesburg
Urban safety’s correlation with holistic quality of life in South Africa’s evolving urban landscape demands comprehensive exploration and understanding.
South Africa’s population, estimated at 58 million in2019 and projected to reach 65 million by 2030 and 70 million by 2043, underscores the significance of quality of life amid rising urbanization rates.
The exploration of the relationship between elements of the built environment, like urban safety, and quality of life is pivotal in shaping present and future urban developments in South Africa.
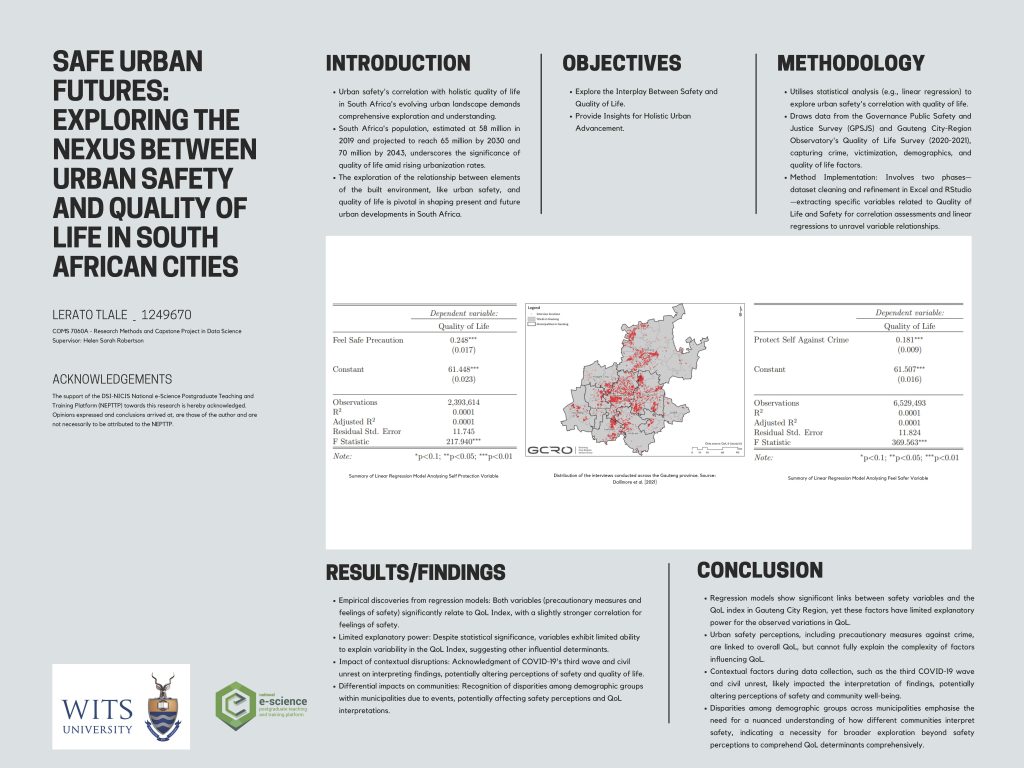